In the current political, social, economic, and technological context, data is essential for business applications and anti-fraud purposes, as it’s the cornerstone of both AI algorithms used for financial fraud prevention and marketing strategies that can significantly affect corporate profitability. Are you a venture manager active in a competitive domain where innovation is at new heights? Do you want to improve your brand’s reputation and streamline your management’s decision-making process? If so, you need to make use of your informational sources.
Data can give you insights into the developments happening in your sector, define the KPIs necessary for the continuous expansion of your business identity, help with operational efforts optimization, and lay the foundation of your financial strategies. However, the viability of your corporate plans will be closely linked to the fraud-prevention measures you apply. How can data assist in anti-fraud applications? For starters, merging multiple data sources will identify anomalies and patterns that cannot typically be detected in real-time by traditional, rule-based fraud-prevention applications.
The gathered informational data will be used to train algorithms and NLP models to identify transactional fluctuations indicating online fraud. The more comprehensive the data for training the AI model, the higher its accuracy and adaptability to market conditions. The accuracy of AI-based fraud-prevention applications is generally above 95%, while their F1 score, which represents the harmonic average between accuracy and sensitivity, often exceeds 0.9. However, the efficiency of AI fraud prevention tools will depend on data enrichment operations.
What Is the Importance of These Operations?
In AI-based fraud-prevention applications, automated data enrichment represents the process by which first-party informational data can be merged with the one received from third-party sources. The result of data enhancement is the expansion of the information sets used by AI-based tool algorithms for training purposes. The more complex the data set, the more accurate and effective the fraud-detection software will identify fraudulent financial activities. Data enhancement is relatively straightforward, as it involves the collection of transactional records, customer usage patterns, and previous service interactions, which are combined with third-party digital collections obtained from external sources.
Above all else, AI models need data. However, this data may come from countless sources. Digital information can be anything from geo-location data, credit history, and informational inquiries subtracted from public databases. Once the necessary digital information is acquired, it can be converted into a standard format understood by the deep learning algorithms, sorted for duplicate content, and integrated via schema matching. The third-party information data can be subtracted using integration interfaces and data integrator connectors, and its analysis process could be streamlined by storing it on centralized data lakes. Moreover, enrichment of the gathered data can be made possible through ETL pipelines.
What Are the Main Advantages of Data Enrichment?
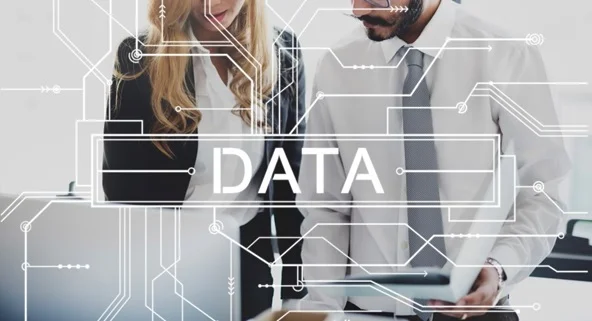
Digital data enhancement can improve the overall accuracy of AI algorithms and positively affect the real-time analytical capabilities of the NLP models utilized by the fraud-detection software. In other words, by merging multiple sets of data at once, the fraud detection application used by your company will be more accurate, as it will detect potential fraud attempts before they have the potential to test the vigilance of your workers. A more precise fraud-prevention program will increase the operational efficiency of your internal teams and have a positive effect on your service’s profitability. Plus, AI-based fraud-detection applications can more easily adapt to market development and new patterns in online fraud techniques.
The use of comprehensive enriched data will add context to raw statistical points, which will enhance the link between the information gathered from first- and third-party digital sources. In other words, enriched data can put together the information presented from different environments and utilize it to increase the accuracy of anomaly detection algorithms and reduce the false positives of the used application. Data enrichment can also significantly improve the predictive machine learning models utilized by fraud-detection programs, help with the segmentation of transactional data, and foster the generation of detailed reports that can give insights into the most commonly used fraud techniques circulating in your activity sector.
What Are the Financial Benefits of AI Fraud-Detection Systems?
Artificial Intelligence has undergone exponential growth in recent years, which has implicitly increased the sophistication of financial fraud methods used by bad actors. Do you want your firm to stay in business without suffering reputational losses or pauses in productivity? If that’s the case, the most cost-effective solution available to you is to use AI-based fraud detection solutions. AI systems can identify fraudulent activities in real-time, and their accuracy rate is significantly higher than traditional rule-based detection systems.
AI fraud detection tools can utilize their adaptive learning models to prevent fraud attempts before they can impact the financial stability of your venture. Plus, since they can streamline internal tasks, AI tools offer reduced long-term operational costs while being scalable as your business expands. AI-based fraud-prevention tools will increase the security of your firm’s transactional data and help you comply with the financial and data protection regulations active in your sector. Consequently, this will increase the number of customers willing to access your services and result in a higher ROI.
A Simple Choice with Far-Reaching Consequences
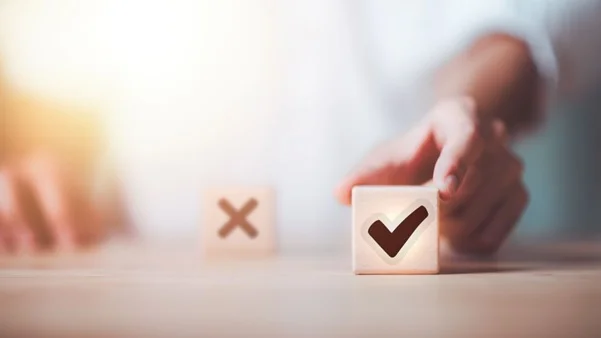
Constant data aggregation from multiple first- and third-party sources will improve the overall efficiency of the AI models utilized to detect fraud attempts. Data enrichment will facilitate the real-time monitoring of your firm’s recorded transactional data as the informational package obtained from the combined data sources will contribute to the creation of a model capable of self-improvement and adaptability to specific market vectors. Enriched data will improve the accuracy of risk scores calculated for each financial transaction and reduce the false positives of the utilized fraud prevention tool.
In other words, enriched data can provide more context to the gathered informational data and increase the accuracy and cost-effectiveness of the utilized tools. AI-based fraud-prevention applications can stop fraud attempts before they can affect business productivity, and they are more scalable than rule-based traditional fraud detection methods. Are such tools necessary? In the context of the current digital landscape, the answer is yes. High-quality AI-based fraud detection applications are required for corporate financial stability, and they represent the only solution through which firms can mitigate the economic risks of their professional activity.
If you found this article helpful, feel free to explore our blog more here.